Project
The fundamental questions will be investigated for different important complex networks such as biological, brain, process, infrastructure, transport and reaction networks. The vision of the group is to become a world class centre of research of understanding governing principles of naturally occurring systems and design of the next generation of man-made networks.
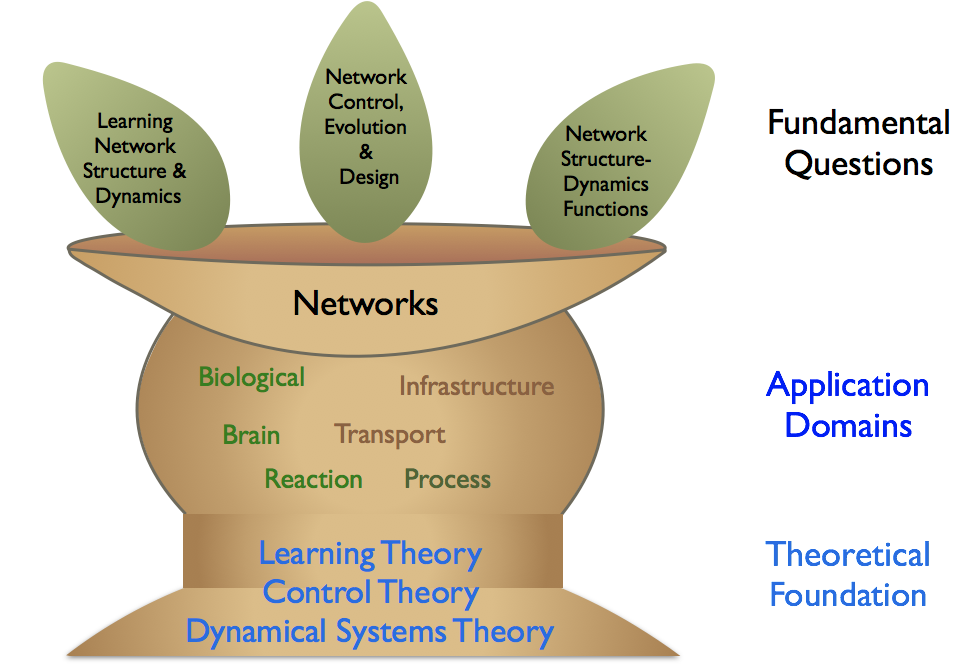
The Network Systems Learning, Control and Evolution Group focuses on three thematic areas: (i) Learning networks structure and dynamics from data, (ii) Network structure-dynamics functions, and (iii) Network control, evolution and design. Particularly, we are interested in investigating questions in these thematic areas arising in biological, brain, infrastructure, transport, chemical reaction and process networks.
Biological networks
Biological networks arising in cellular processes, bacteria colonies, ecological systems, food web etc. are an important class of networks in complex network sciences. These networks have gone through many cycles of evolutionary selection and hence, they exhibit properties such as robustness, resilience, flexibility, and self-organization. A holistic understanding of design principles governing biological networks under different conditions is a quintessential question in network science. The unravelling of design principles of biological networks has implications in understanding the origin of diseases and therapy. Further, understanding these principles can help design next-generation man-made networks. In the last decade, high throughput data at different levels from genomics to fluxomics have opened up new opportunities for understanding the underlying cellular processes in normal and disease conditions. In this vertical, we are interested in developing approaches for learning multilayer biological networks (integrated biological networks for a context) from multi-omics and literature data. Further, we are interested in studying different network properties such as robustness, resilience, flexibility and their roles in normal and disease conditions. These context-specific network models and their properties will be exploited to design drug, therapeutic and diet interventions using network and control theory.
Human Brain Networks
Human cognition is associated with a complex sequence of interactions between multiple networks in the brain. The static organization of brain networks is captured as a map of the neural connections called the connectome. A major objective of brain connectomics study, is that it may lead to a formal mapping of connectivity to brain dynamics. In this context, a significant gain from the network perspective study of the brain is that it may lead to a deeper understanding of the relation between the (structural and functional) connectivity and mental disorders. For example, cross network interactions between three brain networks (salience network, central executive network and the default mode network) are weaker in children with ADHD disorders, and the degree of weakness (of interactions) is actually correlated to the severity of the disorder. New imaging techniques (for e.g. diffusion tensor imaging) allow researchers to compute the structural connectivity of the brain.
In this vertical, we are interested in developing new analytics techniques for characterizing human brain dynamics. Particularly, we work on controllability properties of individual brain regions and of entire brain networks and apply our finding for precision mental health.
Process Networks
Processes have been traditionally represented in the systems theory framework as inter-connections of units involving chemical reactions, mass and/or energy transfer and other physico-chemical phenomena. Each unit is usually described by a set of differential equations, which then leads to either an input-output or a state-space representation. With the assumption of linear time-invariance (of the system), the models are further simplified. A holistic model for the process is then developed by fusing the sub-models in a mathematically formal manner. This approach has served as the backbone of all simulation, design, control and monitoring methodologies and applications. In recent times, significantly so in the last decade, network representations of processes have assumed prominence for important reasons. Network or graphical representations, apart from other features, enable the user to represent variables as both causes and effects, in contrast to the a priori distinction that the user is compelled to respect in conventional representations. Process networks for dynamical systems can be broadly classified into four different classes of graphical representations, namely, those that represent undirected-total, undirected-direct, directed-total and directed-direct connectivity. The classes of directed-total and directed-direct are essentially the causal versions of their undirected versions. In several applications, clearly, it is the directional class that is of interest since they provide the causal information, which in turn is critical to fault diagnosis, performance monitoring, process innovations, etc. Of utmost interest and challenge is the identification of directed-direct class of representations since it is free of confounding, or mediating ambiguities.
In this work, we are interested in addressing the two fundamental problems associated with optimal input design for determining the structure of the directed dynamical network. Furthermore, we are interested in developing process network representations from first-principles from data and its role in process performance monitoring.
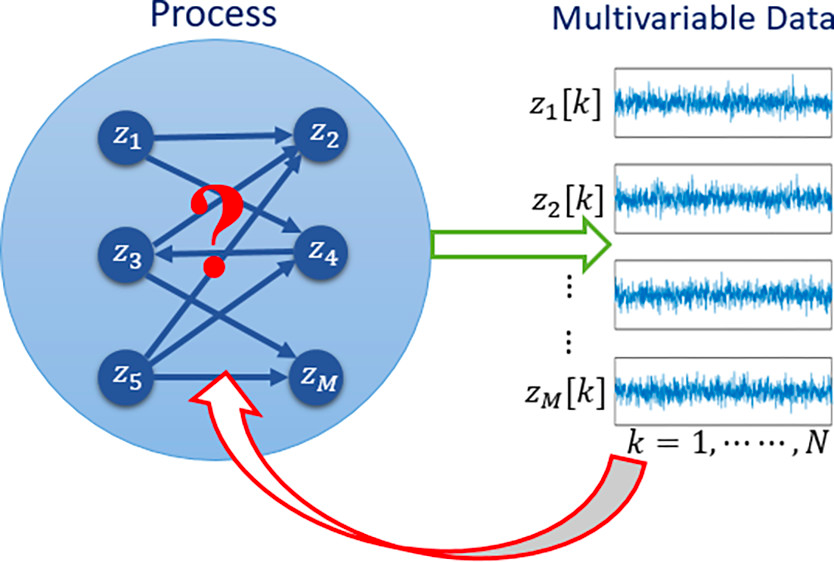
Infrastructure Networks
Infrastructure networks arising out of engineering or man-made technology and organization are central to the safe and productive operation of societies, organizations, health and economy. Examples of these include the water distribution network, traffic networks and health care networks. These networks do not necessarily operate in isolation, but are rather coupled with the natural (climate) and social systems. A key objective of this proposal is to develop predictive understanding of the combined climate (stressors), engineered (stressed), and social (impacted) systems, i.e., the network of networks. This is obviously quite challenging given that the theory of understanding a single class of networks is yet to fully mature. Given the strong correlation that exists within and across the stressors, stressed and impacted systems, network representation can be a plausible unified framework to represent knowledge in the aforementioned systems. However, the knowledge acquired from the network-based representation and analyses should be supplemented and complemented with the information that aids our understanding about individual systems. For example, in case of climate related stressors, while networks can give dynamical understanding of climate oscillators and teleconnections; data sciences, statistics and local scale physics still play pivotal roles in generation of actionable insights. In case of infrastructure systems, both built and natural, the network-based knowledge needs to be complemented and supplemented with data acquisition, data representation, information management, real time data ingestion and offline data analyses. Finally, in impacted systems, while network-based solution frameworks can bridge the key knowledge gaps in our understanding of societal resilience, this knowledge may not be sufficient enough to inform the policy, econometrics and incentive structures for decision making.
Specifically, we are interested in two aspects of these interconnected networks, namely (i) developing network representations for the combined network of networks from the use of physical principles and Big Data and (ii) studying the resilience of the combined system when it is subject to unprecedented hazards.
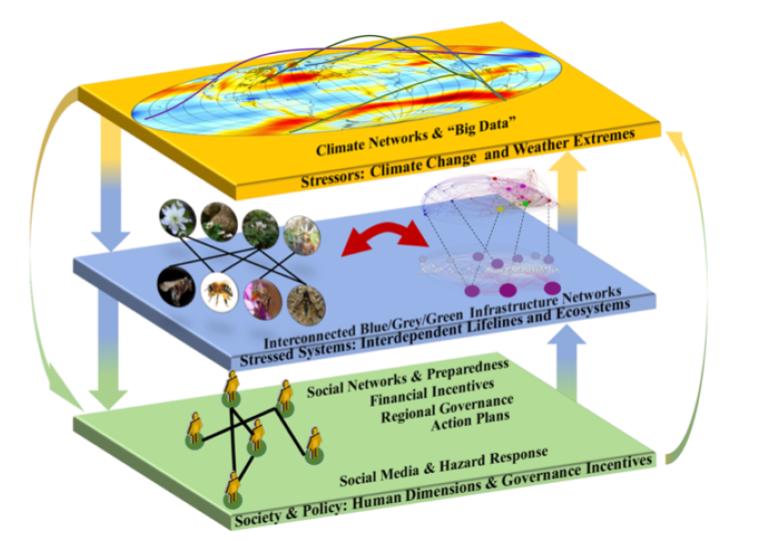
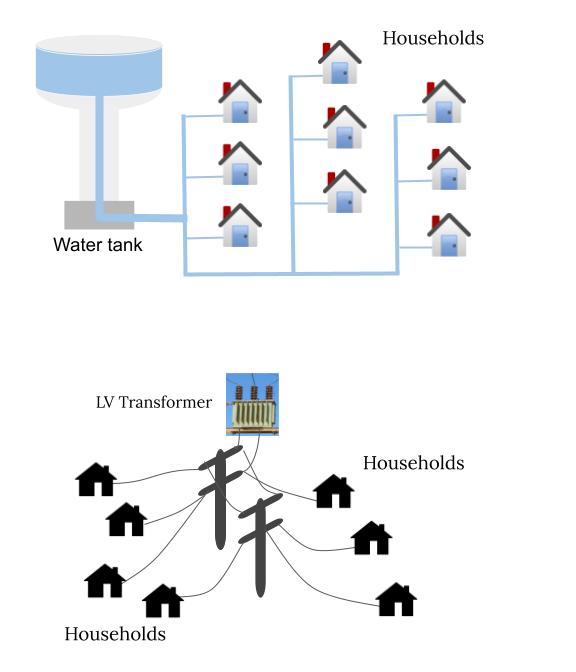
Chemical Reaction Networks
Chemical reactions play an important role in chemical engineering, chemistry, biology and biotechnology due to its central role in different chemical transformations. Understanding of chemical reaction networks has implications in different applications ranging from unraveling origin of life to maximizing production of desired biopharmaceuticals. Traditionally, chemical reaction networks are analyzed using topology information and restrictive assumptions of mass-action kinetics. However, these networks evolve over time and dynamics play an important role in additions to their topology in exhibiting emergence properties. Further, dynamical network models allow us to investigate network properties and its emergence through its interaction with network structure. Further, these models can be used to improve our ability to predict and manipulate these networks for obtaining desired properties. Hence, it is important to infer network dynamics along with network topology from steady-state and transient (or time-series) data. Furthermore, since biological networks are a network of networks, it is also important to construct dynamic models for all the networks from data such that it captures their interactions and direct and indirect influences on different components of sub-networks. Inferring dynamic networks from data is an iterative process involving the following steps (i) collecting steady-state or time-series data by carefully designed experiments, (ii) inferring dynamic model structure and parameters from these data, and (iii) validating the predictive ability of the identified model. Although these steps are well studied in systems theory, the extension to complex networks is not straightforward. In complex biological networks, measurements for all nodes or links with time are not possible to obtain.
In this vertical, we are interested in developing systematic approaches to identify complex and large-scale reaction networks from dynamics and steady-state data. Particularly, in this project, we are interested in studying the minimum number of measurements required to ensure structural and practical identifiability of chemical reaction networks. Further, we are interested in developing approaches for testing structural and practical identifiability of a given chemical reaction network. Optimal experimental design to distinguish between competitive models will be studied.
Expected deliverables of the research
The mission of this research group is to create a world research and teaching group in areas of network systems learning, control and design. The high quality research work during the project will be disseminated through publications in top-tier journals and conferences. The algorithms and frameworks developed will be packaged as software. Furthermore, the group will train high quality manpowers and organize tutorials, schools, and lectures in areas of network systems learning and control.
Current status
Functional Controllability of Brain Circuits
Human cognition is associated with a complex sequence of interactions between multiple networks in the brain.Recent research has seen import of tools from control theory towards studying controllability properties of brain circuits, and investigating the possibility of restoring or enhancing brain activity using brain stimulation. Our results [1] suggest that network controllability is load and context-dependent (working memory in our context); in other words, functional brain circuits are more difficult to control during complex, compared to simpler, cognitive tasks. Currently we are interested in investigating the development of a core cortical-subcortical saccade network that guides visual attention [1] Cai W, Ryali S, Pasumarthy R, Talasila V, Menon V. Dynamic brain circuits during working memory and their functional controllability. Nature Communications, (In Press)
- Funding: Virtual Network “Center for Big Data and the Brain for Precision Mental Health”, Source: IUSSTF. Duration: 2021-2023.
- Participating Institutes: Stanford Medicine, IIT KGP, Clemson University.
Hybrid Intelligent Systems in Driving Autonomy
With a viewpoint of reducing accidents owing to human errors, boosting passenger safety, optimizing travel time and fuel consumption, increasing traffic throughput and enhance road space utilization, there is considerable interest in developing autonomous vehicles in the last decade. The project aims to develop an intelligent system for the maneuver of on-road autonomous vehicles. We propose a hybrid intelligent framework using tools from statistical learning theory and predictive control techniques. Through this project, we attempt to integrate the learning-based AI and model-based predictive control strategies for improving the driving performance of autonomous vehicles. The specific objectives of the project are listed below: • A hybrid framework of Nonlinear model predictive controller and Artificial Intelligence-based agent. • A Control algorithm for on-road connected and autonomous vehicles using the hybrid framework. The idea of developing a hybrid intelligent controller for connected and autonomous vehicles is a kickstart to the long-term goal of implementing these technologies in a real-life vehicle. The proposed control algorithms will be validated on an indigenously developed experimental testbed.
- Funding Agency: SERB, PI: Ramkrishna Pasumarthy, co-PI Nirav Bhatt
- PhD Student: Subhadeep Kumar
Network Design for Controllability:
The problem of control of complex network systems offers challenges mainly due to the size of the network, first in terms of the energy required to control a large network and second computational aspects in choosing a set of driver nodes that ensure controllability, or designing a network topology that minimizes the control effort. Further these networks evolve with time and might change their structure with time, where traditional methods may prove insufficient to guarantee desired network functionality. Our current research is on (i) proposing algorithms that design an optimal Network topology that maximises controllability [1] and (ii) optimising driver nodes in case of temporal networks.
- Principal Investigator: Ramkrishna Pasumarthy
- Collaborator: Rachel Kalaimani
- PhD Student: Valli SM
- [1] MV Srighakollapu, R Kalaimani, R Pasumarthy , “Optimizing average controllability of networked systems” 58th IEEE Conference on Decision and Control (CDC), 2066-2071, 2019
Learning Conserved Networks from Data
Reconstruction of network topology from data is one of the important problems in network science. Networks can be visualized as a chain of interconnected physical or abstract edges through which different entities flow from one node to other nodes. Furthermore, flows through the edges in such networks are conserved at each incident node. This property of conservation leads to a set of networks which is referred to as conserved networks in this work. Flow of reaction flux from one metabolites to another in metabolic biological networks, flow of commodity (such as power, gas, water) flows from a source to a set of consumers in distribution networks, and flow of information or money flow from a sender to a set of receivers in communication or economical networks are examples of conserved networks [1]. The information of network topology is important for better understanding of these networks and also for monitoring, and optimization of them. In this project, it is proposed to develop algorithms for learning exact network topology for conserved networks from data, and partial information.
- Principal Investigator: Nirav Bhatt and Shankar Narasimhan
- Collaborators: Ramkrishna Pasumarthy
- PhD student: Jayadev Pappu, Master Students: Akshara S. (IDDD-Data Science), Arsh Bawa (IDDD-Data Science)
- [1] Pappu, Satya Jayadev, Nirav Bhatt, Ramkrishna Pasumarthy, and Aravind Rajeswaran. “Identifying topology of low voltage distribution networks based on smart meter data.” IEEE Transactions on Smart Grid 9, no. 5 (2017): 5113-5122
Input (Experimental) Design for Network Reconstruction
Designing excitation signals and determining other experimental requirements for network identification (NI) is a prime issue of concern since it can provide answers to the quantity and quality of data (excitation and signal-to-noise ratio) that is required for identifying a reliable network model. Solutions to these fundamental problems not only prove useful in situations where interventions are possible, but also to those cases where only observational data is available since they allow us to determine for a given data, the type of network that is identifiable.
Two questions of interest:
For a given causality (directionality) measure or graph encoding and a given causal network size, what is the optimal input (in terms of excitation frequencies and power) that allows us to determine the structure of the directed dynamical network? A natural method for structure learning is the delay estimation method as proposed in Kathari and Tangirala (2019). Given such a method, then the question is what input would be optimal? How do the input excitation requirements scale up with the network size? Further, do the requirements depend only on certain graphical properties of the network?
The study is restricted to linear time-invariant systems and using computational / simulation models.
Principal Investigator: Arun K. Tangirala
Collaborator: Lakshminarayanan, S. (NUS)
Student: Pranav Agarwal (M.S. Scholar)
References:
- S. Kathari and A.K. Tangirala. A Novel Causality Method for Reconstruction of Process Topology in Multivariable LTI Dynamical Systems. 58th Proceedings of SICE 2019, pp. 199-204.
- Paul R. Rosenbaum. Causal inference in randomized experiments. In: Design of Observational Studies. Springer Series in Statistics. Springer, Cham, pp. 23-72, (2020).
Developing process network models using graph ML methods
Traditional models of linear systems have relied on signal representations as functions of time / frequency / space using appropriate basis. These representations of signals are generally suitable for uniform sampling. However, there exist numerous applications that involve irregular sampling and variations in spatial directions render these representations difficult. In the recent decade, graph signal processing and graph machine learning theories have been propounded as more powerful and unifying frameworks for signal and system representations, especially for data-driven methods. In this project, we are specifically interested in the following objectives
Objectives:
Develop methods for reconstructing process models (network / traditional) using the graphical representations of signals.
Develop metrics for performance monitoring of multivariable systems based on the process models developed in (1) for handling missing data and event-based sampling scenarios.
The study is restricted to linear time-invariant systems.
- Principal Investigator: Arun K. Tangirala
- Collaborator: Lakshminarayanan, S. (NUS)
- Student: Bala Rajesh Konkathi (Ph.D. Scholar)
References:
A. Ortega, P. Frossard, J. Kovacevic, J.M.F. Moura and P. Vandergheynst (2018). Graph Signal Processing: Overview, Challenges, and Applications, Proceedings of the IEEE, 808-828.
J. Mei and J. M. Moura (2017). Signal processing on graphs: Causal modeling of unstructured data. IEEE Transactions on Signal Processing, 65, 8, 2077–2092.
Reconstruction of Biological Networks from Omics and Literature Data
Tissue or disease specific whole cell models are useful for understanding biological processes in a particular tissue type or disease condition, and discovering novel therapeutic strategies. Furthermore, the integrated whole cell models can be used for establishing structural-functional relationships. A context-specific integrated biological model is built by integrating information from different sources. One of the important question is how to integrate literature data and multi-omics data optimally for building a reliable context-specific model. In this project, the main objective is to develop a framework for building a context-specific integrated biological network from data. Currently, we are working on (i) method for integrating signalling-metabolic and regulatory-metabolic networks from literature data for cancer, and (ii) method on developing a context-specific models using natural language processing for causal gene-gene regulatory networks
- Principal Investigator: Nirav Bhatt
- Collaborators: Swagatika Sahoo, Raghunathan Rengaswamy, G K Suraishkumar
- PhD students: Pavan Kumar S, Subasree S.
- Masters Students: Abhishek Sureddy(IDDD-Data Science), Sai N. (DD-BT), Renuka Devi M. (DD-BT)
Structural and Practical Identifiability of Chemical Reaction Networks
For reaction networks, a priori structural identifiability addresses the question of determining whether it is possible to recover the unknown parameters uniquely from a proposed model structure using the noise-free data. Identifiability analysis of reaction networks involves two related questions: the reaction rate identifiability and the structural rate identifiability. For reaction networks, we have used the theory of reaction variants and invariants [1] to simplify the problem of determining structural identifiability. For non-isothermal reaction networks, (described by non-rational functions), we have proposed a method to test identifiability for systems with non–rational functions using Pade approximations and differential algebra [2]. In spite of development of structural identifiability test, the current approaches cannot be applied to complex or large chemical reaction networks due to computational complexity. In this project, we are interested in developing efficient theoretical and algorithmic approaches for (i) checking structural and practical identifiability in reaction systems, (ii)performing reparamterization of unidentifiable structures to make it identifiable, and (iii) estimating kinetic parameters of large-scale reaction systems using steady-state and dynamic data.
- Principal Investigator: Nirav Bhatt and Sridharakumar Narasimhan Collaborators: Radhakrishnan Mahadevan (University of Toronto) and Bayu Jayawardhana (University of Groningen)
- PhD student: Pavan Kumar S.
- Masters Students: Dhruvi Shah(IDDD-Data Science)
- [1]Varghese A, Narasimhan S, Bhatt N. A Priori Parameter Identifiability in Complex Reaction Networks. IFAC-PapersOnLine. 2018 Jan 1;51(15):760-5.
- [2]Jain R, Narasimhan S, Bhatt NP. A priori parameter identifiability in models with non-rational functions. Automatica. 2019 Nov 1;109:108513.
Collaborations
International Collaborations
-
Prof. Lakshminarayanan Samavedham, Department of Chemical and Biomolecular Engineering, National University of Singapore link
-
Prof. Paul van Den Hof, Department of Electrical Engineering, TU Eindhoven, Netherlands.link
-
Prof. Bayu Jayawardhana, Faculty of Science and Engineering, University of Groningen, The Netherlands link
-
Prof. Vinod Menon, Stanford Cognitive and Systems Neuroscience Laboratory, Stanford University, USA, link
-
Prof. Radhakrishnan Mahadevan, Chemical Engineering and Applied Chemistry University of Toronto, Canada link
Societal impact
The questions that we wish to answer in biological networks have important implications for health, ageing and drug discovery. From the brain network and specifically from the mental health point of view, there are very few researchers working from the data point of view. Lack of adequate data collection infrastructure restricts diagnosis to subjective signs and symptoms. This project will give us access to work on real time data collected from Stanford medicine, and the outcome will equip Indian researchers and clinicians with better understanding and techniques and funding mechanisms to diagnose mental health disorders. Infrastructure networks such as power, water and traffic networks play an important role in our lives. This is all the more relevant given the thrust towards electrification and piped water supply for all. Analysis of robustness, adaptation, resilience and stability properties in these networks will help us understand them better and design and evolve networks.
Sustenance statement
For sustenance of the group, we will actively seek extra mural funding from national and international funding organizations for establishing centre of excellence in Network systems The group will actively seek CSR support and funding from industry related to application domains , especially power, water, infrastructure networks, pharmaceutical industries.
Technical/ Scientific Progress
New work done in the project
Several projects are currently ongoing in the pCoE for Network Systems Learning, Control and Evolution.
A brief description of work achieved in the different projects as follows:
Functional Controllability of Brain Circuits:
In this project, system identification, network science, stability analysis, and control theory tools are used to probe functional circuit dynamics during working memory task performance. The results show that dynamic signaling between distributed brain areas encompassing the salience (SN), fronto-parietal (FPN), and default mode networks can distinguish between working memory load and predict performance. Network analysis of directed causal influences suggests the anterior insula node of the SN and dorsolateral prefrontal cortex node of the FPN are causal outflow and inflow hubs, respectively. Network controllability decreases with working memory load and SN nodes show the highest functional controllability. Our findings reveal dissociable roles of the SN and FPN in systems control and provide novel insights into dynamic circuit mechanisms by which cognitive control circuits operate asymmetrically during cognition. The work is published in Nature Communication.
Hybrid Intelligent Systems in Driving Autonomy:
In this project, a model primarily focused on the powertrain of an internal combustion engine vehicle with control-by-wired sub-systems is developed. The powertrain considered in this work comprises a spark-ignition engine, a single-stage lockup torque converter, a double pinion planetary gear set, a pulley type push belt CVT, and associated hydraulic systems. The planetary gear set is modelled as a differential-algebraic system with switching dynamics. The algebraic variable changes when the system dynamics switch during a shift in gear mode from neutral to drive or reverse and vice-versa. Existing models are extended with rotational and actuation dynamics for the other components. Finally, the individual models are aggregated following a modular approach. We study the developed approach on examples real-life scenarios to analyse the aggregated model’s response and determine the model’s reliability. The work is submitted to a journal.
Network Design for Controllability:
The project deals with understanding the concept of controllability on networks. First, We derive conditions for structural controllability of temporal networks that change topology and edge weights with time. We further address two important issues; the first is optimizing the number of driver nodes to ensure the structural controllability of the temporal network. The second is to characterize the maximum reachable subspace when there are constraints on the number of driver nodes.
We address the problem of identifying a network topology of a networked system for maximizing a controllability measure, the average controllability under constraints on the number of links in the network. We consider networked systems consisting of subsystems with higher-order discrete-time linear time-invariant dynamics. We show that the average controllability is a monotone increasing supermodular function of a set of links in the networked system. The work in these projects is published in IEEE Transactions on Control of Network Systems, IEEE Transactions Control Systems Letter, and Systems & Control Letters
Learning Conserved Networks from Data:
In this work, we have worked on solving topology identification and completion problems with respect to topology of conserved networks from flow data. For topology identification and completion, the proposed methodologies can handle both noise-free and noisy data using Principal Component Analysis (PCA) and its variants. Further, it is proven that the identified topology is identical to the true topology under certain conditions. The algorithms developed based on the proposed methodologies are shown to be of polynomial time complexity. The simulation experiments are performed on different networks including standard distribution networks to demonstrate the generality of the solutions and their robustness to handle noisy data. The work on the topology identification is under revision for IEEE Transactions on Network Science and Engineering while the work on topology completion is published in Journal of Complex networks.
The other problem that we have been working on is topology verification. We investigate the different types of errors that could possibly occur in the reporting of a topology. We propose steps to detect, identify, isolate and rectify each of the errors using noise-free flow data. We further extend theoretical finding to handle noisy data and develop an approach to handle the topology verification problem in an online manner. Currently, we are working on a manuscript to submit to either Networked Systems - 9th NECSYS 2022™ or MTNS 2022.
Reconstruction of Biological Networks from Omics and Literature Data:
In this project, we investigate two lines of problems:
- related to developing context-specific models for biological networks, and
- reconstructing network topology or relationships between different biological entities using natural language processing from literature data.
- Developing context-specific models for biological networks: In the first direction, we investigate the fundamental problem of thresholding for building context-specific models from GEMS. In this work, we propose a novel thresholding algorithm, called {Localgini}, that utilizes Gini coefficients to derive gene-specific thresholds using omics data. Localgini is implemented as a pre-processing step to derive core reactions for MeMs. Context-specific models for NCI-60 cancer cell lines are built using distinct MeMs to demonstrate the use of Localgini. The Performance of Localgini is compared to thresholding methods, LocalT2 and StanDep. Localgini derived models recover the most number of housekeeping reactions compared to other thresholding methods. Moreover, the derived models are self-consistent. Localgini gives transcriptomic support to the low expression reactions to include them in the core reaction list. This work is submitted to ISMB 2022. Furthermore, we have been working on building genome-scale models using transcriptomics and literature data for ovary, ovarian cancer and Polycystic ovary syndrome (PCOS). Here, we have developed a genome-scale model for ovary tissue.
- Reconstructing network topology using natural language processing from literature data: In this work, we develop an automated pipeline for reconstructing biological networks from the literature data using pre-trained NLP (Natural Language Processing) models such as BERT and BioBERT for a particular context. We apply the developed approach to four data sets to reconstruct different biological networks. We will use this work in extracting context-specific models for different contexts from the literature data.
Structural and Practical Identifiability of Chemical Reaction Networks:
In this work, we investigate approaches to build dynamic reaction models from different kinds of data sets for practical identifiability of chemical reaction networks. The work involves developing approaches for identifying kinetic models of reaction networks from spectral and concentration data for biochemical and chemical reaction systems. In the first project, Two calibration-free methods are proposed which uses prior information about the reaction system but do not require labelled spectral data. The proposed methods use kinetic model structures and/or minimal offline concentration measurements to estimate kinetic parameters directly without the need for time-intensive calibration experiments. The applicability of the proposed methods is illustrated using noisy simulated data from an example reaction system. The research work is submitted for possible publications. In the second project, the incremental model identification approach is developed for biochemical reaction systems. A systematic approach to develop a kinetic model of bioprocess involving a single biomass from concentration data is proposed without imposing any kinetic model a priori. The proposed incremental model identification approach decomposes the model building task into a set of sub-tasks such as determining the yield coefficients and maintenance coefficient, specific growth rate structure identification, and parameter estimation. It is shown that the proposed approach allows us to identify the mechanism of product formation. The proposed approach is applied to microbial production of Hyaluronic acid (HA). The part of work is submitted to a conference.
Infrastructure developments
Several computational facility is developed for high-end work.
Output
The work published or submitted in conferences and journals for different projects are as follows:
Functional Controllability of Brain Circuits: Cai W, Ryali S, Pasumarthy R, Talasila V, Menon V. Dynamic causal brain circuits during working memory and their functional controllability. Nature Communications. 2021 29;12(1):1-6.
Network Design for Controllability:
-
Srighakollapu MV, Kalaimani RK, Pasumarthy R. On Strong Structural Controllability of Temporal Networks. IEEE Control Systems Letters. 2021 Dec 7;6:1861-6.
-
Srighakollapu MV, Kalaimani RK, Pasumarthy R. Optimizing network topology for average controllability. Systems & Control Letters. 2021 Dec 1;158:105061.
-
Srighakollapu MV, Kalaimani RK, Pasumarthy R. Optimizing driver nodes for structural controllability of temporal networks. IEEE Transactions on Control of Network Systems. 2021.
Learning Conserved Networks from Data:
- Pappu SJ, Pasumarthy R, Bhatt N. Predicting unknown directed links of conserved networks from flow data. Journal of Complex Networks. 2021 Dec;9(6):cnab037.
- Pappu SJ, Narasimhan, S., Bhatt N. Learning Conserved Networks from Flows Data, under revision, 2021, preprint
Hybrid Intelligent Systems in Driving Autonomy:
- Kumar, S, Bhatt N, Pasumarthy, S. “Electronically Actuated CVT Powertrain: Part I - Model”, submitted to Vehicle System Dynamics, 2022
- Kumar, S, Bhatt N, Pasumarthy, S. “Electronically Actuated CVT Powertrain: Part II- Simulation”, submitted to Vehicle System Dynamics, 2022
Biological networks: Kumar, PS, Bhatt N, “Localgini: Gini coefficient based thresholding algorithm to build constraint based context-specific metabolic models”, submitted to Intelligent Systems for Molecular Biology 2022 (ISMB 2022)
Structural and Practical Identifiability of Chemical Reaction Networks:
-
Sridhar S, Bhatt N, Suraishkumar GK. Mechanistic insights into ureolysis mediated calcite precipitation. Biochemical Engineering Journal. 2021 Dec 1;176:108214.
-
Kamakshi C, Jayaramana G., and Bhatt N., “Incremental Model Identification of Bio-processes from Data: Application to Microbial Production of Hyaluronic Acid”, submitted to DYCOPS 2022
-
Manokaran, V, Sreeja, S, Narasimhan, S., and Bhatt, N., “Calibration-free semi-supervised machine learning based kinetic modelling using spectral data from flow reactors”, submitted
-
Manokaran, V, Sreeja, R, Narasimhan, S., and Bhatt, N., “Identification of Complex Reaction Systems from Unlabelled Spectroscopic Data in Flow Reactors”, submitted to AIChE Journal
Tutorial session in Indian Control Conference 2020 on “A priori identifiability of chemical, biochemical and biological networks” by Nirav Bhatt and Sridharakumar Narasimhan
A special seminar by Prof M. Vidyasagar, FRS, SERB - National Science Chair and Distinguished Professor, IIT Hyderbad on “SUTRA for modelling COVID-19 in India and elsewhere” was organized.
Mobility
Visits planned for PI, co-PIs, international collaborators and students (both inbound and outbound)
-
PI/co-PIs are planning to attend SICE of Japan 2022, 9th NECSYS 2022™, MTNS 2022, DYCOPS 2022.
-
With the SICE society of Japan, we are planning to organize a special Session in either the conference of SICE of Japan 2022 or Indian Control Conference 2022.
-
In MTNS 2022, we are planning to organize a tutorial on “Analysis of Chemical Reaction Networks” with Faculty members from the Gronigen University.
-
Visits planned for International collaborators: Profs Jacqueline Scherpen, Bayu jayawardhana, Umesh vaidya, Paulo Tabuada will visit for short duration during summer or december during ICC 2022. Student: Pavan Kumar S (research stay at Prof. radhakrishnan mahadevan at University of Toronto).
Relationship
Industrial Engagement
We would like to work with industries in areas of network systems control and process automation. We have worked with Pfizer on the analysis of reaction systems data. We are actively in discussion with industries such as pfizer, Indian Oil Ltd to implement some of our research findings.
University Engagement
Prof. Sridharakumar Narasimhan and Nirav Bhatt have written an Indo-Australian propposal on the infectious disease prevention and control with the Curtin University. Currently, we are discussing with them for highering joint doctoral students in areas of infection diseases prevention and control using network control theory. Further, we are working on establishing a joint-degree program in areas of systems and control with European universities.
Updates
Relevant Updates
pCoE is going to organize Indian Control Conference 2022 at IIT Madras.